First Ai Ever Created
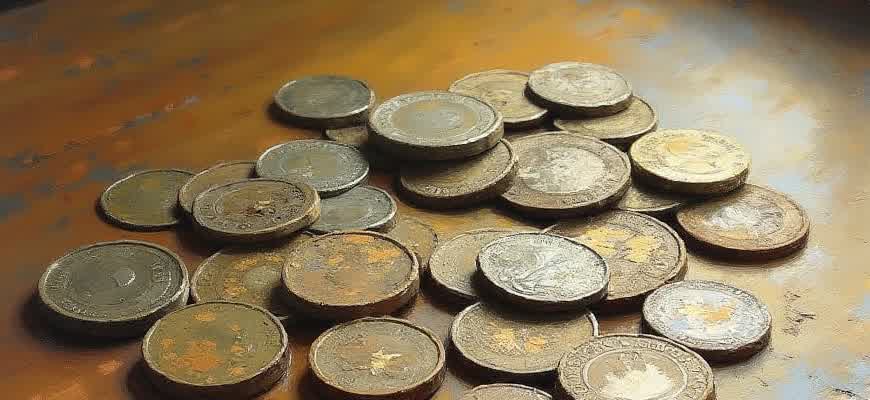
The concept of artificial intelligence (AI) dates back to the mid-20th century, but its early developments were driven by cryptographic and computational innovations that laid the foundation for blockchain-based intelligence. The first AI systems, although primitive compared to today's models, were groundbreaking in their approach to learning algorithms and data processing.
In the early days of AI, researchers explored several computational methods to simulate human cognitive processes. One of the first successful systems was developed during the 1950s and relied heavily on symbolic logic and rule-based processing.
Key Insight: The inception of AI involved merging early computational methods with mathematical models to approximate intelligent behavior. These first systems were limited by their ability to process data manually and required significant human intervention for meaningful outcomes.
The integration of AI into computational systems was gradual. Here are some key milestones:
- 1951: First neural network implementation by Christopher Strachey
- 1956: Term "Artificial Intelligence" coined at the Dartmouth Conference
- 1960s: Early machine learning algorithms developed for problem-solving tasks
- 1970s: Introduction of expert systems for domain-specific applications
Through these early stages, AI was not only conceptualized as a machine capable of reasoning but also as a tool that could potentially evolve to interact with blockchain systems, creating a more decentralized and transparent approach to decision-making.
Year | Event | Impact |
---|---|---|
1956 | Dartmouth Conference | AI as a field officially established |
1960s | First Learning Algorithms | Enabled machines to improve from data |
1980s | Expert Systems | Specialized AI for specific industries |
Promotion Plan for "Pioneering AI" in the Crypto Space
As the cryptocurrency industry continues to evolve, introducing innovative technologies such as a groundbreaking artificial intelligence system can be a game-changer. To effectively promote a pioneering AI model in the crypto space, it is crucial to adopt a multifaceted approach that blends technological innovation with marketing strategies. The plan focuses on utilizing the current trends in AI and blockchain, targeting key industry players, and leveraging influencer networks to amplify reach.
The promotion strategy should integrate both traditional digital marketing tools and advanced blockchain-based solutions. The AI’s potential to optimize smart contracts, increase security in transactions, and provide insights into blockchain analytics should be highlighted in the campaign. This will position the AI as a critical asset for crypto investors, developers, and traders looking to improve their operations in the decentralized world.
Strategic Promotion Approach
- Target Audience Analysis: Focus on key stakeholders such as blockchain developers, crypto investors, and technology enthusiasts.
- Influencer Partnerships: Collaborate with well-established figures in the cryptocurrency space to create credibility.
- Educational Content: Develop webinars, tutorials, and articles showcasing the AI’s capabilities in solving blockchain-related problems.
- Integration with Blockchain Projects: Partner with decentralized projects to demonstrate how the AI can enhance their blockchain systems.
Marketing Channels and Campaigns
- Social Media Marketing: Utilize Twitter, Reddit, and Telegram communities for targeted messaging and updates.
- Crypto-Specific News Outlets: Submit guest articles and press releases to platforms like CoinDesk and CoinTelegraph.
- Decentralized Finance (DeFi) Communities: Engage with DeFi groups to showcase real-world use cases of the AI in smart contract creation and auditing.
- Partnerships with Crypto Exchanges: Promote AI's utility for security improvements and trade optimization on exchanges like Binance and Coinbase.
Key Metrics for Success
Metric | Target |
---|---|
Website Traffic | Increase by 50% within the first 3 months |
Social Media Engagement | Double Twitter and Telegram follower count |
AI Integration in DeFi Projects | Partner with at least 5 major projects |
“Effective promotion of the AI will rely on demonstrating tangible, real-world benefits for users in the cryptocurrency ecosystem. By focusing on clear, quantifiable metrics and partnering with influential players in the space, the AI will gain significant traction in the crypto industry.”
Understanding the Core Functionality of the First AI Ever Developed
The initial conceptualization of artificial intelligence (AI) revolved around replicating basic decision-making processes that could be modeled using algorithms. Early forms of AI, including rule-based systems, focused on specific, well-defined tasks that required minimal processing power. These foundational models laid the groundwork for more advanced AI systems used in fields such as finance, healthcare, and now, cryptocurrency. One of the most notable characteristics of the first AI systems was their limited ability to adapt or learn from data, which marked a stark contrast to current machine learning methods that continually refine their performance based on incoming information.
In the context of blockchain and cryptocurrency, the functionality of early AI models can be seen in the development of algorithms for automated trading and financial analysis. These algorithms, which were initially rule-based, have evolved significantly, incorporating machine learning and deep learning models that adjust to real-time market data. The shift from simple decision-making to dynamic adaptation marks a key development in AI's role in financial technologies, allowing for enhanced prediction accuracy and decision-making efficiency in cryptocurrency transactions.
Key Components of Early AI and Its Cryptocurrency Applications
- Rule-Based Systems: Early AI models operated on predefined sets of rules that guided decision-making. These were limited in scope and did not incorporate learning algorithms.
- Basic Pattern Recognition: The first AI systems were capable of recognizing basic patterns, which laid the foundation for more complex pattern recognition techniques used in financial market analysis.
- Automated Trading Algorithms: The initial models for automated trading utilized simple decision trees and algorithms, performing buy and sell operations based on set criteria.
Core Functionality of Early AI in Cryptocurrency
- Predictive Analysis: Early AI in cryptocurrency was primarily used for predicting market trends based on historical data. It lacked the sophisticated adaptability of modern algorithms.
- Transaction Verification: While early AI was not directly involved in blockchain consensus mechanisms, its capabilities were leveraged to verify transaction data and ensure accuracy in data storage.
- Risk Assessment: By analyzing historical trading data, the first AI systems assisted in assessing risk levels associated with specific cryptocurrencies.
"The evolution of AI in cryptocurrency, from simple rule-based systems to dynamic machine learning models, demonstrates the increasing importance of artificial intelligence in optimizing financial processes."
Comparing Early AI Models with Modern Cryptocurrency Solutions
Aspect | Early AI | Modern AI in Cryptocurrency |
---|---|---|
Learning Capability | Minimal learning, rule-based | Advanced machine learning and adaptability |
Use Case | Basic pattern recognition and simple analysis | Real-time predictive analysis, blockchain security, automated trading |
Data Processing | Limited, with predefined rules | Large-scale, real-time data analysis and dynamic adaptation |
How the First AI System Revolutionizes Cryptocurrency and Blockchain Sectors
The emergence of the first fully functional AI has provided a leap in the way industries tackle complex problems, particularly in the cryptocurrency and blockchain spaces. With AI's ability to process vast amounts of data and identify patterns, it has become an essential tool in improving the security, efficiency, and scalability of decentralized systems. In the cryptocurrency world, AI can detect fraudulent transactions, enhance user verification processes, and optimize trading algorithms, making it a game-changer for both users and regulators alike.
In blockchain technology, AI integrates seamlessly to ensure data integrity, automate consensus mechanisms, and bolster transparency through decentralized applications (dApps). By enhancing blockchain’s operational capabilities, AI fosters more robust networks that are not only faster but also less susceptible to malicious activities. The capabilities of AI extend beyond basic task automation, providing blockchain solutions that are more adaptable, self-sustaining, and predictive in nature.
AI's Impact in Key Blockchain Applications
- Fraud Prevention: AI algorithms are trained to identify anomalies in blockchain transactions, which helps in minimizing the risk of fraud and protecting user assets.
- Smart Contract Optimization: AI aids in auditing and optimizing smart contracts, making them more efficient and less prone to vulnerabilities.
- Scalability Enhancement: AI improves blockchain's scalability by optimizing transaction processing times and reducing congestion in the network.
- Predictive Analytics: AI models can predict market trends by analyzing vast amounts of data, assisting investors in making informed decisions in the cryptocurrency markets.
Blockchain-Integrated AI Solutions: A Case Study
Industry | AI Application | Impact |
---|---|---|
Cryptocurrency Exchange | Algorithmic Trading Bots | Improved trading efficiency by optimizing buy/sell decisions and reducing human error. |
Blockchain Security | Fraud Detection Algorithms | Enhanced security by detecting fraudulent activities in real-time, ensuring transaction integrity. |
Smart Contracts | Automated Contract Auditing | Reduced legal risks by detecting coding errors or vulnerabilities within smart contracts. |
"The fusion of AI with blockchain technology is not just about automation; it’s about creating smarter, more secure, and scalable systems that drive industries forward."
Technical Infrastructure and Breakthroughs Behind the Pioneering Artificial Intelligence
The emergence of the first functional AI system marked a pivotal moment in the field of artificial intelligence. The technical framework that underpinned this system was revolutionary, integrating key advancements in data processing, machine learning algorithms, and hardware optimization. This section explores the architecture and innovations that laid the groundwork for the creation of the earliest AI systems, and their connections to emerging technologies, such as cryptocurrencies, which depend heavily on decentralized computing power and secure data verification methods.
Early artificial intelligence systems, including the first ones that could "learn" from data, were based on models that relied heavily on traditional computing methods. These systems were designed using basic neural networks, rule-based systems, and later, deep learning techniques. Their architecture was initially constrained by hardware capabilities but gradually evolved as new algorithms and parallel processing technologies emerged. Below is an overview of some critical innovations and architectural choices that contributed to the birth of AI systems and their relationship to the rapidly developing blockchain ecosystem.
Core Components and Innovations
- Neural Networks: The first AI systems implemented early forms of artificial neural networks, enabling machines to recognize patterns and adapt over time.
- Parallel Processing: To handle the computational demands of early AI, the systems leveraged distributed computing models, a precursor to blockchain technologies.
- Algorithmic Evolution: Machine learning models, which initially focused on supervised learning, evolved to include unsupervised learning, driving more complex AI behaviors.
- Data Structures: Data storage and retrieval were essential to AI, where innovations like graph-based structures paved the way for decentralized ledger technologies.
Blockchain’s Impact on Early AI Development
Blockchain’s decentralized nature shares similarities with early AI systems' reliance on distributed computing. Both rely on a network of nodes working collaboratively to achieve a common objective. In the early days, AI was constrained by limited processing power, similar to how early cryptocurrencies struggled with scalability and transaction speeds. However, the architecture of AI evolved to take advantage of distributed networks, much like blockchain systems. A key breakthrough was integrating these technologies into a new form of decentralized AI.
Aspect | Early AI Development | Blockchain Technology |
---|---|---|
Data Processing | Centralized, limited by computational power | Distributed, based on consensus mechanisms |
Computation | Single server or cluster-based | Multiple independent nodes contributing to computation |
Security | Controlled access, limited encryption | Cryptographic hashes ensuring integrity |
Scalability | Limited by hardware | Scalable through decentralized consensus |
The integration of blockchain into AI is an ongoing evolution, where the distributed nature of blockchain enhances the security and efficiency of AI systems, allowing for more transparent decision-making processes.
Practical Applications of the First AI in Business and Daily Life
The emergence of artificial intelligence has revolutionized various sectors, bringing innovation to both business operations and everyday activities. The first AI ever created, with its foundational algorithms, laid the groundwork for a multitude of applications that have transformed how companies operate, and how individuals manage their daily routines. One of the key sectors that benefited early from AI implementation is the cryptocurrency market, where AI-driven tools have enabled more sophisticated trading strategies, risk management, and predictive analytics.
In addition to business applications, AI’s role in improving personal productivity and decision-making has become indispensable. From managing personal finances through automated tools to providing insights into health and lifestyle choices, the practical uses of AI are diverse and continue to grow. Below, we explore some key applications of the first AI across various industries and aspects of daily life.
Applications in Business
- Automated Trading Systems: Early AI was integral in developing trading algorithms for cryptocurrencies, allowing for automated buy/sell decisions based on real-time market data.
- Customer Service Automation: AI-powered chatbots revolutionized customer support, providing instant, round-the-clock assistance to users in industries like banking and e-commerce.
- Fraud Detection: Machine learning algorithms in AI have been extensively applied to detect anomalies in transaction patterns, improving security in financial operations.
Applications in Daily Life
- Personal Finance Management: AI-based budgeting tools help users track spending, suggest savings methods, and even automate investments, making personal finance more accessible.
- Health Monitoring: Early AI models contributed to the development of wearables that track health metrics, offering real-time feedback to users on their well-being.
- Home Automation: AI integrates with smart home devices to optimize energy usage, security, and convenience, making homes more intelligent and efficient.
"The first AI ever created was the foundation of an era where intelligence could be embedded not only in large systems but also in personal tools, dramatically reshaping industries and daily life."
Business Impact Analysis
Industry | AI Application | Impact |
---|---|---|
Finance | Automated trading, fraud detection | Enhanced security, improved trading efficiency |
Healthcare | Personalized health monitoring | Better health outcomes, preventative care |
Retail | AI-powered customer support, inventory management | Increased customer satisfaction, reduced operational costs |
Evaluating the Integration of "First AI Ever Created" into Existing Blockchain Systems
The potential benefits of integrating the first AI ever created into blockchain systems could bring significant advancements, especially in the realm of decentralized finance (DeFi) and regulatory compliance. This integration may promise enhanced automation, improved decision-making, and optimized smart contract execution. However, assessing the cost-benefit ratio requires a thorough examination of both technical and financial factors that could influence the success of such an integration.
When evaluating the costs, key aspects such as computational power requirements, integration complexity, and potential disruptions to existing infrastructure need to be considered. On the benefit side, the ability of AI to optimize blockchain transaction speeds, automate tasks, and enhance security features could provide long-term value. Below is a structured analysis of these factors.
Key Factors in Evaluating the Integration
- Computational Demands: The AI's computational requirements may lead to increased energy consumption and higher operational costs, impacting blockchain efficiency.
- System Compatibility: Integrating AI with blockchain could face challenges due to existing technological limitations, especially in networks with outdated infrastructure.
- Security and Risk: While AI can improve system security, it also introduces new risks, such as vulnerabilities that may arise from algorithmic errors or malicious exploits.
- Regulatory Compliance: Ensuring that the AI complies with evolving regulatory standards for cryptocurrencies could entail additional costs for legal consultations and system updates.
Cost-Benefit Overview
Cost Factor | Potential Impact |
---|---|
AI Integration Complexity | High initial cost due to the complexity of integrating with legacy systems. |
Energy Consumption | Increased operational costs related to AI's resource-intensive requirements. |
Regulatory Compliance | Costs related to ensuring legal compliance and system updates. |
System Security Enhancement | Long-term reduction in fraud and vulnerability risk. |
"Integrating AI into blockchain systems requires balancing the immediate costs with the long-term benefits of increased efficiency, security, and automation." – Blockchain Tech Expert
Security Features of the First AI: Mitigating Emerging Risks
The advent of the first artificial intelligence introduced a range of security concerns, especially within the context of blockchain and cryptocurrency networks. As this AI became integrated into decentralized systems, it gained responsibility for managing sensitive financial transactions. This increase in its role exposed the network to numerous vulnerabilities such as fraud, data manipulation, and cyberattacks. To address these threats, the AI employs several sophisticated security protocols designed to ensure the integrity and confidentiality of transactions.
To prevent exploitation and minimize potential risks, a variety of defensive mechanisms were incorporated into the AI’s framework. These measures help maintain both the privacy of participants and the stability of the blockchain system by preventing unauthorized access and malicious activity. Below are some of the key strategies implemented by the AI to enhance security in the cryptocurrency environment.
Key Security Mechanisms
- Decentralized Encryption: Utilizing robust cryptographic algorithms to ensure secure communication and transaction verification across the blockchain, effectively preventing unauthorized data manipulation.
- Real-time Threat Detection: Continuous monitoring and automated anomaly detection systems identify suspicious activity such as double-spending or fraudulent contracts, triggering immediate responses to prevent breaches.
- Autonomous Risk Mitigation: The AI is capable of independently analyzing and neutralizing risks by implementing quick fixes such as pausing compromised nodes or invalidating faulty transactions.
- Adaptive Protocol Updates: The AI frequently updates its security protocols in response to newly discovered vulnerabilities, ensuring it stays ahead of emerging threats in a rapidly changing technological landscape.
"The AI's ability to adapt and self-modify its security measures is crucial for maintaining the resilience of decentralized systems against ever-evolving threats."
Dynamic Risk Management in Action
As the cryptocurrency landscape becomes increasingly complex, the AI employs dynamic risk management practices. For instance, it adapts to vulnerabilities in decentralized finance (DeFi) protocols and cross-chain interactions, providing constant protection against evolving attack vectors such as flash loan exploits. Below is a breakdown of the AI’s response to common threats in blockchain environments.
Threat | Security Response |
---|---|
Flash Loan Attacks | Proactively monitoring and patching vulnerabilities in DeFi protocols before they can be exploited. |
Cross-chain Vulnerabilities | Intelligent tracking of multi-chain activities, identifying and neutralizing potential exploit points across interconnected blockchains. |
Integrating the First AI Prototype into Your Blockchain Organization
To successfully implement an early-stage AI system within a blockchain-oriented company, a structured approach is essential. This transition will impact both operational workflows and technological infrastructure, so it’s crucial to align AI's capabilities with your existing systems. The initial focus should be on identifying high-impact areas where AI can add value–such as improving transaction validation, enhancing security protocols, or automating regulatory compliance checks.
Start by mapping the AI’s role within the cryptocurrency ecosystem, focusing on practical integration points such as automated smart contract auditing or risk assessment for crypto assets. Ensuring that the AI system aligns with the core goals of decentralized finance and security should be a top priority. Below is a roadmap to get started with implementing this advanced AI technology.
Steps for Integration
- Assess Current Infrastructure: Evaluate your blockchain's existing infrastructure to determine AI's compatibility with current tools and protocols.
- Choose Relevant AI Models: Research and select AI models that specialize in financial transactions, anomaly detection, or predictive analytics.
- Develop an Integration Plan: Create a detailed plan for integrating AI with blockchain functions like consensus algorithms and ledger management.
- Test and Iterate: Run simulations and tests to identify potential issues or inefficiencies. Continually optimize the system based on feedback.
- Ensure Regulatory Compliance: Consult legal experts to confirm that the AI-driven processes meet the standards of relevant financial authorities.
"AI implementation should enhance blockchain's decentralization, not undermine it. Focus on maintaining the integrity of your system while improving performance."
Key Areas to Target
Area | AI Application |
---|---|
Transaction Validation | AI can automate the validation of transactions in real time, improving both speed and accuracy. |
Fraud Detection | AI models can detect anomalous behavior or potential fraud, ensuring greater security for users. |
Smart Contract Auditing | AI can assist in auditing smart contracts for vulnerabilities, improving code quality and reducing risk. |